Decentralized AI: The Next Evolution in Artificial Intelligence
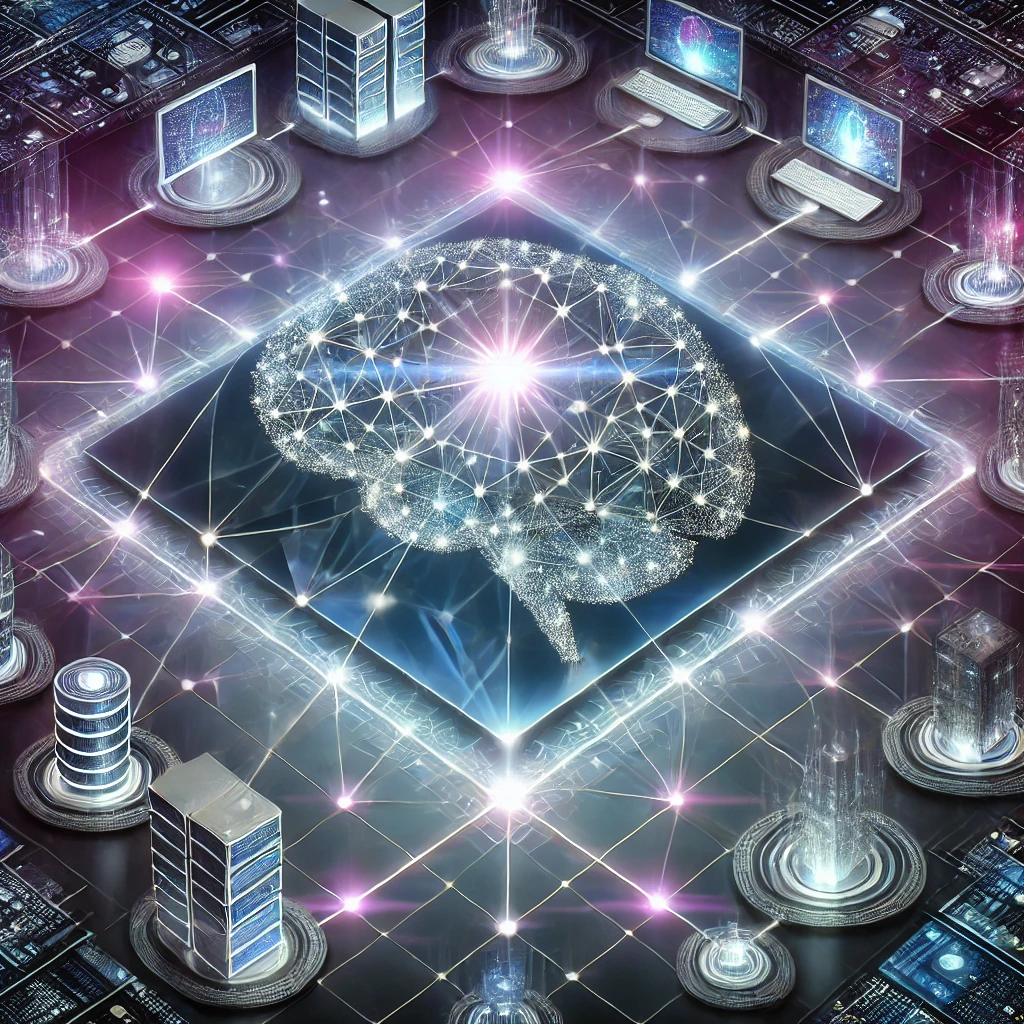
Decentralized AI (dAI) represents a paradigm shift from traditional, centrally managed artificial intelligence solutions, distributing data, compute power, and decision-making across interconnected nodes. As the demand for data privacy, resilience, and inclusivity grows, dAI emerges as a promising avenue to create more equitable and transparent AI ecosystems.
Understanding Decentralized AI
Traditional AI models often rely on centralized repositories of data and compute power. These hubs, controlled by a single entity or a small number of stakeholders, can become bottlenecks—raising concerns about data privacy, single points of failure, and potential bias in decision-making. In contrast, decentralized AI disperses data storage, model training, and inferencing across multiple participants in a network. Each node can contribute resources, while collectively achieving AI capabilities that no single participant could easily replicate alone.
Why This Matters:
- Resilience: With no single point of failure, decentralized systems are more resistant to outages or targeted attacks.
- Data Sovereignty: Individuals and organizations can maintain control over their own data, choosing to share models or insights rather than raw information.
- Collaborative Innovation: dAI encourages a broader pool of contributors, potentially accelerating research and application development.
(Source: “Decentralized & Collaborative AI on Blockchain,” IEEE Communications Magazine, 2021)
Key Drivers Behind Decentralized AI
Growing Concern Over Data Privacy
As data breaches and misuse scandals become more frequent, businesses and consumers alike are wary of centralized data storage. Decentralized AI can employ federated learning techniques—training algorithms on local devices without transferring sensitive data to a central server—thus enhancing privacy while still improving model accuracy. For instance, healthcare providers can train AI models on patient data held securely at local hospitals, contributing to a global model without ever sharing identifiable patient records.
Technological Advancements in Distributed Computing
Progress in distributed ledgers, peer-to-peer networks, and secure multi-party computation enables complex tasks to run efficiently across nodes. Blockchain or other decentralized infrastructures can ensure participants trust one another’s contributions, and secure enclaves or zero-knowledge proofs safeguard sensitive computations. This technical foundation sets the stage for robust, scalable dAI ecosystems.
Regulatory and Ethical Imperatives
Policymakers are increasingly focusing on data protection regulations, fairness in AI decision-making, and anti-monopoly measures. Decentralized AI aligns naturally with these goals—diversifying data sources, preventing control by a single entity, and allowing stakeholders to audit and influence algorithmic behavior.
Real-World Applications and Examples
Healthcare Diagnostics:
Imagine a global network of hospitals collectively training an AI diagnostic tool. Each hospital’s patient data remains on-site, preserving patient privacy. The federated learning algorithm aggregates model parameters from all participants to produce a highly accurate diagnostic model. Patients worldwide benefit from improved healthcare insights without compromising data confidentiality.
Supply Chain Optimization:
In complex supply chains, each participant—farmers, manufacturers, distributors—contributes local data about inventories, transit times, and resource usage. A decentralized AI model can integrate these data points to predict demand, optimize routing, and minimize waste. Because the data never leaves each participant’s control, competitive secrets remain secure, and smaller stakeholders gain a voice in shaping the model’s outputs.
Smart Cities and IoT Networks:
As sensor networks proliferate in urban environments, decentralized AI can process data at the edge—traffic patterns, weather conditions, energy usage—without relying solely on a central server. This reduces latency, enhances resilience, and allows local agencies to govern the data they collect, ensuring more transparent and democratic decision-making.
Practical Considerations for Implementation
- Choosing the Right Infrastructure:
Explore platforms that support distributed computations, federated learning frameworks, and secure data-sharing mechanisms. - Ensuring Data Quality and Integrity:
Decentralized AI systems depend on diverse inputs. Setting standards for data validation and quality control helps maintain robust model performance. - Governance Models:
Establish clear protocols for how nodes join or leave the network, how consensus is reached, and how model updates are managed. Transparent governance structures are essential for building trust.
Actionable Steps and Takeaways
- For Organizations:
Begin by piloting federated learning solutions to integrate insights from multiple departments or partners without pooling raw data. - For Policymakers:
Craft regulations that encourage decentralization’s privacy and fairness benefits without stifling innovation. - For Technologists and Researchers:
Experiment with open-source federated learning libraries and distributed computing tools. Contribute to community-driven standards that guide the responsible use of dAI.
As we stand on the cusp of this new frontier, it's clear that dAI has the potential to reshape the AI landscape. By democratizing access to AI development, enhancing data privacy, and creating new economic models, dAI could usher in a more equitable and innovative era of artificial intelligence.
However, the success of dAI will depend on continued technological advancements, thoughtful governance structures, and widespread adoption by developers and users alike. As this field evolves, staying informed about the latest developments and considering the potential implications for various industries will be crucial for technologists, investors, and policymakers.